
Sentiment Analysis: Evolution Over the Years

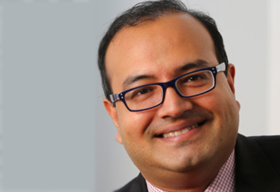
Though originally the scope of sentiment analysis was limited to understanding public perception, over time it has expanded to include feedback and customer views of products and services. The complexity involved in understanding sentiment has also increased exponentially driven by an explosion of online opinion channels, technology-savvy customers, and a generation that lives online to provide & absorb opinions. From a marketing standpoint, I have outlined three changes observed over time.
Complexity in Data Management
For decades, the data sources used to analyze sentiments were limited, it was mainly the opinion expressed through newspapers, emails or others. Many times sentiments were gauged and documented by means of customer interaction with the banking agent directly. Closed groups and third-party surveys were occasionally used not only to understand brand perception, but also a reaction to new product launches or services. Over the years, these data sources have grown exponentially, right from text-based responses on online forums and media to video, as well as voice-based responses. Likewise, the percentage of people expressing an opinion through various channels have also increased tremendously.
Managing large volumes of data is more important than ever. To aid in analysis, data needs to be validated and sources authenticated, to remove any Bot-generated data & duplicates, and segmented to help classification. There is also the prevalence of tremendous amount of real-time data which needs to be collated and managed effectively. Like any analytics engine, sentiment analysis also depends on assigning quantitative values to categorize responses and using them to arrive at insightful information. Collating, categorizing, and assigning values to data is an important step in aiding analysis, one that cannot show results without effective data management.
Expanding Analysis Scope
While originally sentiment analysis was used to understand brand perception, its scope has increased to include viewpoints and reactions to a bank's services and products. In the current banking technology landscape of shorter product release cycles and feature enhancements, sentiment analysis can play a powerful role in understanding user reactions to new enhancements, as well as the sunsetting old products or creating new financial products. A few years ago, BBVA used sentiment analysis to understand customer reaction to a bonus feature being
dropped from their financial product and take corrective action. With agile development engines enabling faster product releases and newer features in financial products, inputs provided by sentiment analysis can quickly be used to make the required changes and create the right impact.
Further, some banks have taken this a step further by looking at the sentiment analysis of competitor products to introduce better, as well as newer features that provide competitive differentiation. While this may not act as the determining factor for new features, it can definitely aid in validating assumptions made, while creating newer product features. Trends churned-out by sentiment analysis can further be used to map certain categories of products to particular demographics or create new products for specific customers based on user reactions to competitor products. Better sentiment analysis engines also provide the ability to slice & dice data to arrive at products that enjoy maximum/minimum liking by the customer base. This can also be used to drive focused campaigns aimed at customer acquisition.
An additional extension of sentiment analysis has been to aid customer service agents understand the emotion of a customer and use it to better service resolution. Few players like Cogito have been addressing this space through AI-supported sentiment analysis tools that enable contact center agents to manage customer calls with due emotional connection guided by intelligence available in real-time. Tools such as CallMiner Eureka enable delivering intelligence-at-scale from the voice of the customer, and present transcriptions that are tagged with sentiment, topic discovery, and customer journey mapping across channels. Calabrio One, on the other hand, provides a comprehensive unified suite that empowers agents through tools that not only capture the voice of the customer to deliver insights, but also correlate the sentiment with call metrics and offers measurable business value. Other tools with such scope include Attensity and Clarabridge.
Complexity in Sentiment Analytics
The explosion in online forums and channels has also brought about a drastic change in the expression of opinions. Today, sentiment analysis also has to take into consideration the complexities of customer sentiment brought forward by the tone of comment, metaphors, sarcasm, and context. Traditional analytics engines which merely classify sentiment as positive or negative are no longer sufficient, AI/ML/NLP-driven engines are the need of the hour. The erstwhile engines served only to give an indication of the perception of a brand, and could at times even be misleading, as emotion gauging has been limited.
In such a scenario, AI engines have a better chance of passing through the detailed comments, contextually analyze them and categorize them. An example is the Tone analyzer by IBM Watson, which has the ability to evaluate online customer feedback and categorize the tone effectively. With ongoing human intervention, such engines become better over the course of time. While they may not be able to obviate human intelligence, they will be able to provide inputs on sentiment categorization making the task of human agents easier. Some of these engines are also able to take facial expressions from video data to arrive at the emotion of the customer a feature that has been successfully used by retail organizations to capture emotions at the time of purchase. Further, the ability to respond to negative comments in real-time has become more important than ever. The drastically small life span of social media comments within a particular context also mandates deployment of AI/NLP engine to analyze the nature of opinions/comments and mapping to a human decision maker for taking appropriate action.
Social listening too plays an important role here in getting deeper insights into the customer's perception and possibly a future course of action. Termed social sentiment analysis, it involves measuring and reporting on the tone and emotion behind one's social media mentions/posts. It is very essential to monitor this as not all customers might engage directly with customer service agents or the company in general to convey their satisfaction or dissatisfaction. Social Sentiment Analysis helps prune a company's social media engagement patterns, measure brand perception/reputation over a period of time, as well as deal with social media crisis on time. Such listening need not be only passive, but can also be proactive, wherein insightful feedback can be begotten, at times done before events such as product/service launches, and many others.
Sentiment analysis has moved be-yond capturing brand perception in identifying customer views on banking products and services, driving the creation of features and new financial products by banks. Along with an in-crease in the applicability of sentiment analysis, we are also seeing an exponential rise in complexities involved in capturing and categorizing sentiments. Traditional ways of sentiment analysis no longer hold ground in view of the above findings, applications of AI/ML/NLP concepts are a must in effectively categorizing sentiments and creating competitive differentiation.
Further, some banks have taken this a step further by looking at the sentiment analysis of competitor products to introduce better, as well as newer features that provide competitive differentiation. While this may not act as the determining factor for new features, it can definitely aid in validating assumptions made, while creating newer product features. Trends churned-out by sentiment analysis can further be used to map certain categories of products to particular demographics or create new products for specific customers based on user reactions to competitor products. Better sentiment analysis engines also provide the ability to slice & dice data to arrive at products that enjoy maximum/minimum liking by the customer base. This can also be used to drive focused campaigns aimed at customer acquisition.
An additional extension of sentiment analysis has been to aid customer service agents understand the emotion of a customer and use it to better service resolution. Few players like Cogito have been addressing this space through AI-supported sentiment analysis tools that enable contact center agents to manage customer calls with due emotional connection guided by intelligence available in real-time. Tools such as CallMiner Eureka enable delivering intelligence-at-scale from the voice of the customer, and present transcriptions that are tagged with sentiment, topic discovery, and customer journey mapping across channels. Calabrio One, on the other hand, provides a comprehensive unified suite that empowers agents through tools that not only capture the voice of the customer to deliver insights, but also correlate the sentiment with call metrics and offers measurable business value. Other tools with such scope include Attensity and Clarabridge.
Complexity in Sentiment Analytics
The explosion in online forums and channels has also brought about a drastic change in the expression of opinions. Today, sentiment analysis also has to take into consideration the complexities of customer sentiment brought forward by the tone of comment, metaphors, sarcasm, and context. Traditional analytics engines which merely classify sentiment as positive or negative are no longer sufficient, AI/ML/NLP-driven engines are the need of the hour. The erstwhile engines served only to give an indication of the perception of a brand, and could at times even be misleading, as emotion gauging has been limited.
In such a scenario, AI engines have a better chance of passing through the detailed comments, contextually analyze them and categorize them. An example is the Tone analyzer by IBM Watson, which has the ability to evaluate online customer feedback and categorize the tone effectively. With ongoing human intervention, such engines become better over the course of time. While they may not be able to obviate human intelligence, they will be able to provide inputs on sentiment categorization making the task of human agents easier. Some of these engines are also able to take facial expressions from video data to arrive at the emotion of the customer a feature that has been successfully used by retail organizations to capture emotions at the time of purchase. Further, the ability to respond to negative comments in real-time has become more important than ever. The drastically small life span of social media comments within a particular context also mandates deployment of AI/NLP engine to analyze the nature of opinions/comments and mapping to a human decision maker for taking appropriate action.
Social listening too plays an important role here in getting deeper insights into the customer's perception and possibly a future course of action. Termed social sentiment analysis, it involves measuring and reporting on the tone and emotion behind one's social media mentions/posts. It is very essential to monitor this as not all customers might engage directly with customer service agents or the company in general to convey their satisfaction or dissatisfaction. Social Sentiment Analysis helps prune a company's social media engagement patterns, measure brand perception/reputation over a period of time, as well as deal with social media crisis on time. Such listening need not be only passive, but can also be proactive, wherein insightful feedback can be begotten, at times done before events such as product/service launches, and many others.
Sentiment analysis has moved be-yond capturing brand perception in identifying customer views on banking products and services, driving the creation of features and new financial products by banks. Along with an in-crease in the applicability of sentiment analysis, we are also seeing an exponential rise in complexities involved in capturing and categorizing sentiments. Traditional ways of sentiment analysis no longer hold ground in view of the above findings, applications of AI/ML/NLP concepts are a must in effectively categorizing sentiments and creating competitive differentiation.